
Reiner Lemoine Institut erstellt Elektromobilitätskonzepte für Städte in NRW
31. Januar 2019
Travelling Conferences 2
7. Februar 2019Implementation and validation of an open source model for generating wind feed-in time series (Haas 2019)
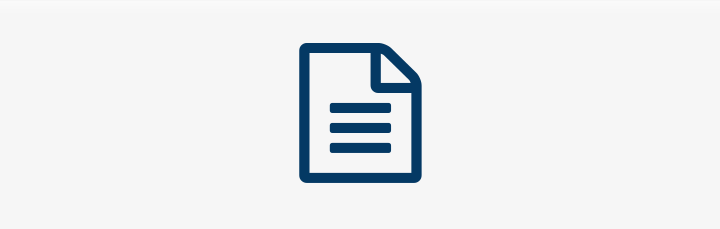
Sabine Haas
Masterarbeit von Sabine Haas, angefertigt im Rahmen ihrer Tätigkeit als studentische Mitarbeiterin im open_FRED-Projekt
Abstract
In this thesis an open source model for generating wind feed-in time series called Windpowerlib is implemented in Python and validated with measured feed-in time series.
A basic version of the Windpowerlib that was developed at the Reiner Lemoine Institute serves as basis of this work. Functionalities like wind speed height corrections, density and power output calculations, power curve smoothing, aggregated power curves and functionalities for the consideration of wake losses are presented, implemented and their e_ect on simulation results evaluated. The validation is carried out with measured feed-in time series of wind parks in Schleswig-Holstein (coastal region) and Brandenburg (inland region) in Germany for the years 2015 and 2016. Moreover, the inuence of weather data on feed-in time series simulations is examined by using two di_erent weather data sets. MERRA-2 data provided by the NASA is compared with open FRED weather data that was especially created for energy systems simulations. Wind farm feed-in can be simulated with a deviation of 4.7 % (inland) and 3.4 % (coastal region) from the measured annual energy output (overestimation) by the Windpowerlib when using open FRED weather data. For MERRA-2 data the deviations are about ten percentage points higher in Schleswig-Holstein (coastal region) and about 26 percentage points higher in Brandenburg (inland region). All generated time series attain strong correlations with the measured time series with Pearson correlation coe_cients of about 0.7 to 0.9 while MERRA-2 data reaches slightly higher correlations compared to open FRED data.
In this thesis an open source model for generating wind feed-in time series called Windpowerlib is implemented in Python and validated with measured feed-in time series.
A basic version of the Windpowerlib that was developed at the Reiner Lemoine Institute serves as basis of this work. Functionalities like wind speed height corrections, density and power output calculations, power curve smoothing, aggregated power curves and functionalities for the consideration of wake losses are presented, implemented and their e_ect on simulation results evaluated. The validation is carried out with measured feed-in time series of wind parks in Schleswig-Holstein (coastal region) and Brandenburg (inland region) in Germany for the years 2015 and 2016. Moreover, the inuence of weather data on feed-in time series simulations is examined by using two di_erent weather data sets. MERRA-2 data provided by the NASA is compared with open FRED weather data that was especially created for energy systems simulations. Wind farm feed-in can be simulated with a deviation of 4.7 % (inland) and 3.4 % (coastal region) from the measured annual energy output (overestimation) by the Windpowerlib when using open FRED weather data. For MERRA-2 data the deviations are about ten percentage points higher in Schleswig-Holstein (coastal region) and about 26 percentage points higher in Brandenburg (inland region). All generated time series attain strong correlations with the measured time series with Pearson correlation coe_cients of about 0.7 to 0.9 while MERRA-2 data reaches slightly higher correlations compared to open FRED data.